4.4. The analytic journey
As in any research method, analyzing collected data is a necessary step in order to draw conclusions. Analyzing qualitative data is not a simple nor a quick task. Done properly, it is systematic and rigorous, and therefore labor-intensive and time-consuming “[…] good qualitative analysis is able to document its claim to reflect some of the truth of a phenomenon by reference to systematically gathered data” (Fielding 1993), in contrast “poor qualitative analysis is anecdotal, unreflective, descriptive without being focused on a coherent line of inquiry.” (Fielding 1993) (Pope et al. 2000, p. 116). Qualitative analysis is a matter of deconstructing the data, in order to construct an analysis or theory (Mortelmans 2009).
The ways and techniques to analyze qualitative data are not easy to describe as it requires a lot of “fingerspitzengefühl” and it is unrealistic to expect a kind of recipe book which can be followed in order to produce a good analysis. Therefore what we present here is a number of hands-on guidelines, which have proven useful to others.
The difficulty of qualitative analysis lies in the lack of standardization and the absence of a universal set of clear-cut procedures which fit every type of data and could be almost automatically applied. Also there are several methods/approaches/traditions for taking the analysis forward (see table). These move from inductive to more deductive, but in practice the researcher often moves back- and forward between the data and the emerging interpretations. Hence induction and deduction are often used in the same analysis. Also elements from different approaches may be combined in one analysis (Pope and Mays 2006).
Different aims may also require different depths of analysis. Research can aim to describe the phenomena being studied, or go on to develop explanations for the patterns observed in the data, or use the data to construct a more general theory (Spencer et al. 2014). Initial coding of the data is usually descriptive, staying close to the data, whereas labels developed later in the analytic process are more abstract concepts (Spencer et al. 2014).
“The analysis may seek simply to describe people’s views or behaviors, or move beyond this to provide explanation that can take the form of classifications, typologies, patterns, models and theories (Pope and Mays 2006, p. 67).”
The two levels of analysis can be described as following:
- The basic level is a descriptive account of what was said (by whom) related to particular topics and questions. Some texts refer to this as the “manifest level” or type of analysis.
- The higher level of analysis is interpretative: this is the level of identifying the “meanings”. It is sometimes called the latent level of analysis. This second level of analysis can to a large degree be inspired by theories.
The selected approach is part of the research design, hence chosen at the beginning of the research process.
In what follows we describe a generic theoretic process for qualitative data analysis.
Figure: Conceptual representation of the analytic journey of qualitative data with an inductive approach
Each theoretical approach adds its own typical emphases. The most relevant approaches are described in next section. These steps could also be useful in the processing of qualitative data following a system thinking method [ADD crossrefs].
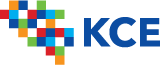
Step 0: Preparing the data for analysis
Independent of the methodological approach, a qualitative analysis always starts with the preparation of the gathered data. Ideally, to enable accurate data analysis the recorded information is transcribed. A transcript is the full length literal text of the interview. It often produces a lot of written text.
Good quality transcribing is not simply transferring words from the tape to the page. The wording is only part of the message. A lot of additional information is to be found in the way people speak. Tone and inflection, timing of reactions are important indicators too. With experienced observers and note-takers, a thematic analysis of the notes taken during the interviews could be used as a basis for analysis of the “non-verbal” communication.
Transcribing is time consuming and costly. The research team should consider in advance the question "who should do the transcribing”? Resources may be needed to pay an audio typist, a strategy usually more cost effective than a researcher. Be aware that “typists” are often unfamiliar with the terminology or language used in the interviews or focus groups which can lead to mistakes and/or prolong the transcribing time.
It may not be essential to transcribe every interview or focus group. It is possible to use a technique known as tape and notebook analysis, which means taking notes from a playback of the tape recorded interview and triangulating them with the notes taken by the observers and note-takers. However, bias can occur if inexperienced qualitative researchers attempt tape and notebook analysis. It is certainly preferable to produce full transcripts of the first few interviews. Once the researcher becomes familiar with the key messages emerging from the data tape analysis may be possible. Transcripts are especially valuable when several researchers work with the same data.
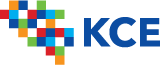
Step 1: Familiarization
Researchers immerse themselves in the data (interview transcripts and/or field notes), mostly by reading through the transcripts, gaining an overview of the substantive content and identifying topics of interest (Spencer et al, 2014). Doing this, they get familiar with the data.
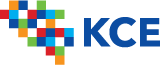
Step 2: Coding the data - Construction of initial categories
By reading and re-reading the data in order to develop a profound knowledge of the data, an initial set of labels is identified. This step is very laborious (especially with large amounts of data). Pieces of text are coded, i.e. given a label or a name. Generally, in the qualitative analysis literature, “ data coding” refers to this data management. However data coding refers to different levels of analysis.
Here are some commonly used terms (Paillé and Muchielli, 2011):
Label:
Labeling a text or part of a text is the identification of the topic of the extract, not what is said about it. “What is the extract about?” The labels allow to make a first classification of the documents/ extracts. They are useful in a first quick reading of the corpus.
Example: “Familial difficulties”
Code:
The code is the numerical/truncated form of the label. This tool is not very useful in qualitative data analysis.
Example: “Fam.Diff.”
Theme:
The theme goes further than the label. It requires a more attentive lecture.
“What is the topic more precisely?”
Example: “Difficulties to care for children”
Statement:
Statements are short extracts, short syntheses of the content of the extract. “What is the key message of what is said?”, “What is told?”
The statement is more precise than the theme because it resumes, reformulates or synthetizes the extract. They are mainly used in phenomenology.
Example: The respondent tells that she has financial difficulties because she has to spend time and money to take care of her children.
Conceptualizing category:
Conceptualizing categories are the substantive designations of phenomena occurring in the extract of the analyzed corpus. Hence, this approaches theory construction.
Example: “Parental overload”
These types of coding terms are generally more specific to certain types of qualitative data analysis methods (Paillé and Muchielli, 2011).
By coding qualitative data, meanings are isolated in function of answering the research question. One piece of text may belong to more than one category or label. Hence there is likely to be overlap between categories. Major attention should be paid to “rival explanations” or interpretations about the data.
For further detailed information on coding qualitative data:
Saldaña J. The coding manual for qualitative researchers. 2nd edition ed. London: Sage Publications; 2013.
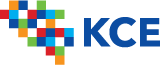
Step 3: Refine and regroup categories
In a third step the categories are further refined and reduced by being grouped together. “While reading through extracts of the data that have been labelled in a particular way, the researchers assesses the coherence of the data to see whether they are indeed ‘about the same thing’ and whether labels need to be amended and reapplied to the data” (Spencer et al. 2014a), p. 282).
Word processors or software for qualitative data analysis [LAK1] will prove to be very helpful at this stage.
[LAK1]Add crosslink vers section process book existante
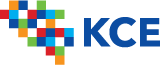
Step 4: Constant comparison
During the analysis the researcher might (as a third step) constantly compare the constructed categories with new data, and the new categories with already analyzed data. This results in a kind of inductive cycle of constant comparison to fine tune categories and concepts arising from the data. NB: In the particular case of focus groups, separate analyses have to be performed on data gathered “within-focus group” and continuously compared “between focus group”. This is also an iterative process.
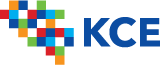
(Step 5): New data collection
New data collection could also be necessary to verify new point of views or insights emerging from the analysis.
Before moving to the more interpretive stage of analysis, the researchers may decide to write a description for each subtheme in the study (Spencer et al., 2014).
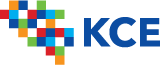
Step 6: Abstraction and interpretation
“Taking each theme in turn, the researcher reviews all the relevant data extracts or summaries, mapping the range and diversity of views and experiences, identifying constituent elements and underlying dimensions, and proposing key themes or concepts that underpin them. The process of categorization typically involves moving from surface features of the data to more analytic properties. Researchers may proceed through several iterations, comparing and combining the data at higher levels of abstraction to create more analytic concepts or themes, each of which may be divided into a set of categories. Where appropriate, categories may be further refined and combined into more abstract classes. Dey (1993) uses the term ‘splitting’ and ‘slicing’ to describe the way ideas are broken down and then recombined at a higher level – whereas splitting gives greater precision and detail, slicing achieves greater integration and scope. In this way, more descriptive themes used at the data management stage may well undergo a major transformation to form part of a new, more abstract categorical or classificatory system” (Spencer et al., 2014, p. 285). At this stage typologies can be created.
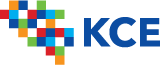
Step 7: Description of the findings and reporting
Laurence.Kohn Tue, 11/16/2021 - 17:41Findings can be presented in a number of ways, there is no specific format to follow.
When writing up findings issued from interviews or texts qualitative researchers often use quotes. Quotes are useful in order to (Corden and Roy 2006):
- Illustrate the themes emerging from the analysis.
- Provide evidence for interpretations, comparable to the use of tables of statistical data appearing in reports based on quantitative findings.
- Strengthen credibility of the findings (despites critics argue that researchers can always find at least one quote to support any point they might with to make).
- Deepen understanding. The actual words of a respondent could sometimes be a better representation of the depth of feeling.
- Enable voice to research participants. This enables participants to speak for themselves and is especially relevant in a participatory paradigm.
- Enhance readability by providing some vividness and sometimes humour: Braking up long passages of text by inserting spoken words, could help to keep the reader focused, but there could be a danger in moving too far towards a journalistic approach.
Ideally, quotes are anonymous and are accompanied by a pseudonym or description of the respondents. For example, in a research about normal birth, this could be: (Midwife, 36 years). There are however exceptions the rule of anonymity, e.g. stakeholder interviews, in which the identity of the respondent is important for the interpretation of the findings. In that case the respondent should self-evidently be informed and his agreement is needed in order to proceed.
Also in terms of lay out quotations should be different from the rest of the text, for example by using indents, italic fond or quotation marks. Quotes are used to strengthen the argument, but should be used sparingly and in function of the findings. Try to choose citations in a way that all respondents are represented. Be aware that readers might give more weight to themes illustrated with a quotation.
When the research is conducted in another language than the language of the report in which the findings are presented, quotes are most often translated. “As translation is also an interpretive act, meaning may get lost in the translation process (van Nes et al.), p. 313)”. It is recommended to stay in the original language as long and as much as possible and delay the use of translations to the stage of writing up the findings (van Nes et al.).
KCE practice is to translate quotes only for publications in international scientific journals, but not for KCE reports. Although KCE reports are written in English, inserted quotes are in Dutch or French to stay close to the original meaning. The authors should pay attention to the readability of the text and make sure that the text without quotes is comprehensive to English speaking readers.
So far, this general a-theoretic procedure reflects what in the literature is called the general inductive approach for analyzing qualitative data. It does not aim at the construction of theories, but the mere description of emerging themes. It provides a simple, straightforward approach for deriving findings in the context of focused research questions without having to learn an underlying philosophy or technical language associated with other qualitative analysis approaches (Thomas, 2006).